Natural Language Processing in Data Analytics
As we navigate the era of data-driven decision-making, one key player has rapidly emerged at the forefront of technological advancement: Natural Language Processing (NLP). A subset of artificial intelligence, NLP aims to allow computers to understand, interpret, and produce human language in a manner that's both meaningful and valuable. But how does NLP fit into the vast world of data analytics?
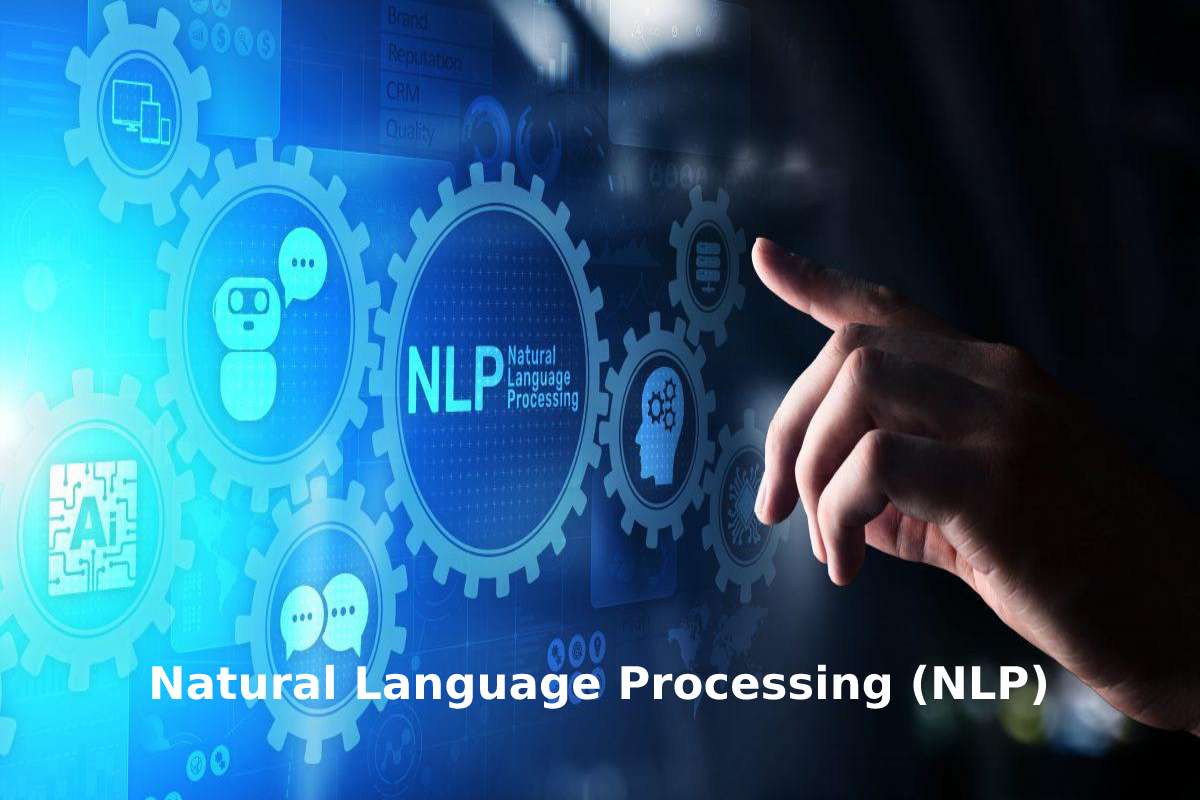
What is Natural Language Processing?
At its core, NLP is a fusion of computer science, artificial intelligence, and linguistics. It enables machines to read, decipher, and make sense of human language, ultimately allowing them to respond in a way that's comprehensible to humans.
NLP in Data Analytics: Why Does It Matter?
- Automated Data Entry and Cleaning: One of the most time-consuming steps in data analytics is data entry and cleaning. With NLP, large volumes of unstructured text data can be processed, analyzed, and cleaned automatically.
- Enhanced Data Visualization: Through NLP, data analysts can generate insightful visual representations based on textual data. This helps in quicker decision-making and trend spotting.
- Sentiment Analysis: For businesses, understanding public sentiment towards their products or brand is crucial. NLP allows for automatic sentiment analysis, gauging public perception from reviews, comments, and more.
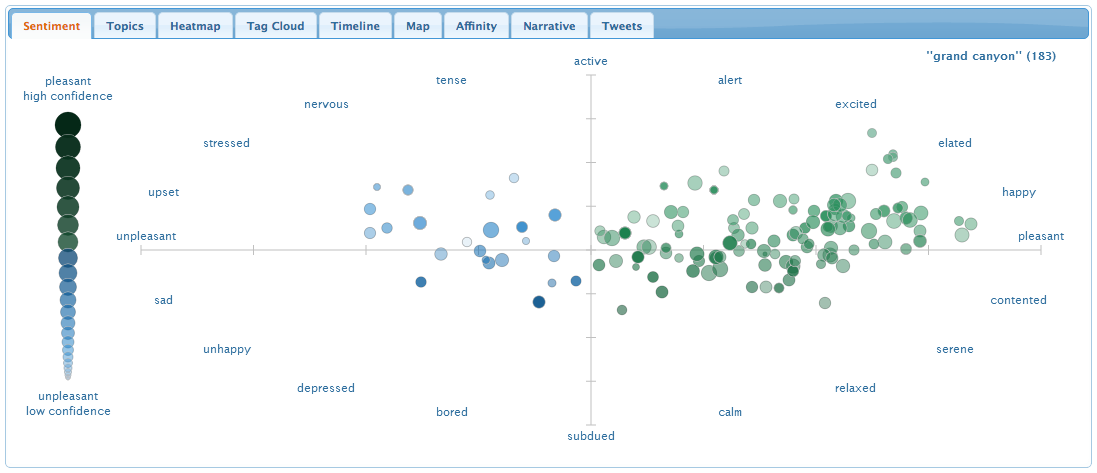
Real-World Applications of NLP in Data Analytics:
- Customer Support Automation: Many businesses are employing chatbots powered by NLP to handle basic customer queries, making the support process more efficient.
- Market Research: Firms can analyze vast amounts of textual data from social media or online reviews to gain insights about market trends or product reception.
- Healthcare: Medical professionals can use NLP to quickly sift through patient records, research papers, or notes, improving diagnosis and treatment processes.
The Future of NLP in Data Analytics:
The current trajectory of NLP suggests a future where human-machine interaction becomes seamless. As NLP algorithms become more advanced, the accuracy and depth of analysis will improve, paving the way for:
- Personalized Content Delivery: Based on user preferences, behaviors, and feedback, systems can generate content tailored to individual users.
- Advanced Predictive Analysis: Using textual data, NLP can enhance predictive models, forecasting future trends or behaviors with better accuracy.
- Real-time Translation and Transcription: Whether it's business meetings or global conferences, NLP will enable instant translations and transcriptions, breaking down language barriers.
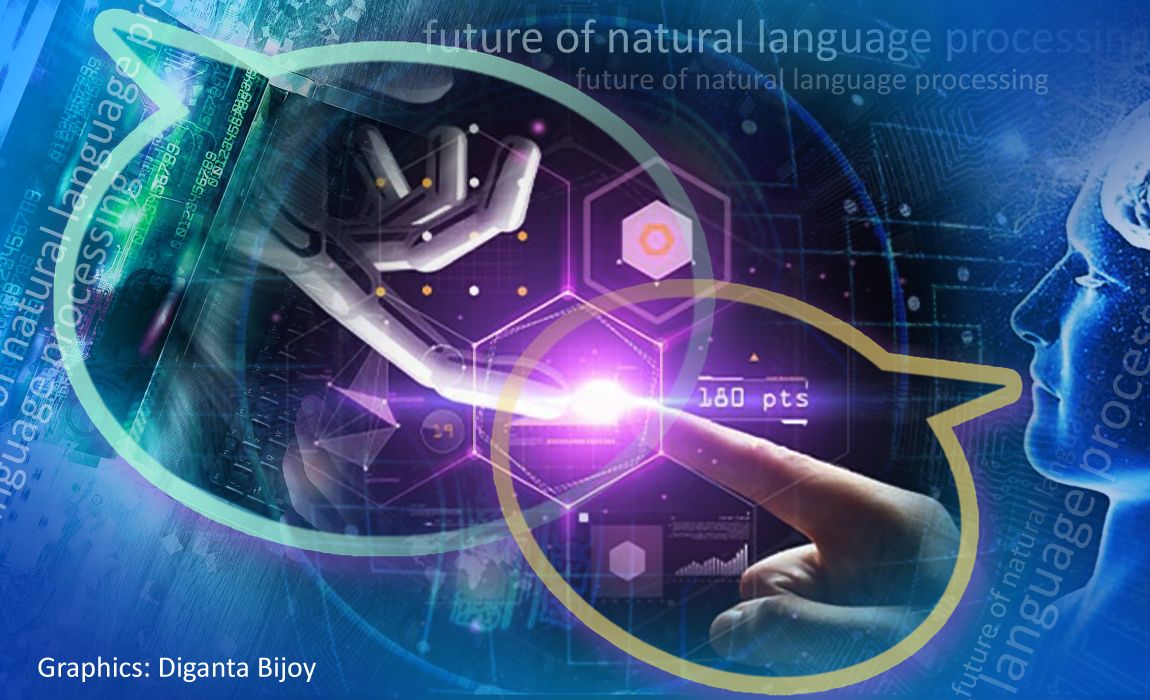
Challenges in NLP for Data Analytics:
Despite the tremendous potential, NLP faces certain challenges:
- Sarcasm and Ambiguity: Human language is riddled with nuances, sarcasm, and ambiguity, making it tough for machines to always grasp the intended meaning.
- Cultural Context: Words and phrases can have different meanings based on cultural contexts, which can lead to misinterpretations.
- Computational Complexity: Advanced NLP requires significant computational power, which might not always be readily available.
Conclusion:
NLP stands as a testament to the strides we've made in bridging the gap between human communication and machine understanding. As it continues to integrate deeper into data analytics, businesses and researchers stand to gain immensely, benefiting from enhanced efficiency, deeper insights, and more meaningful interactions with technology.